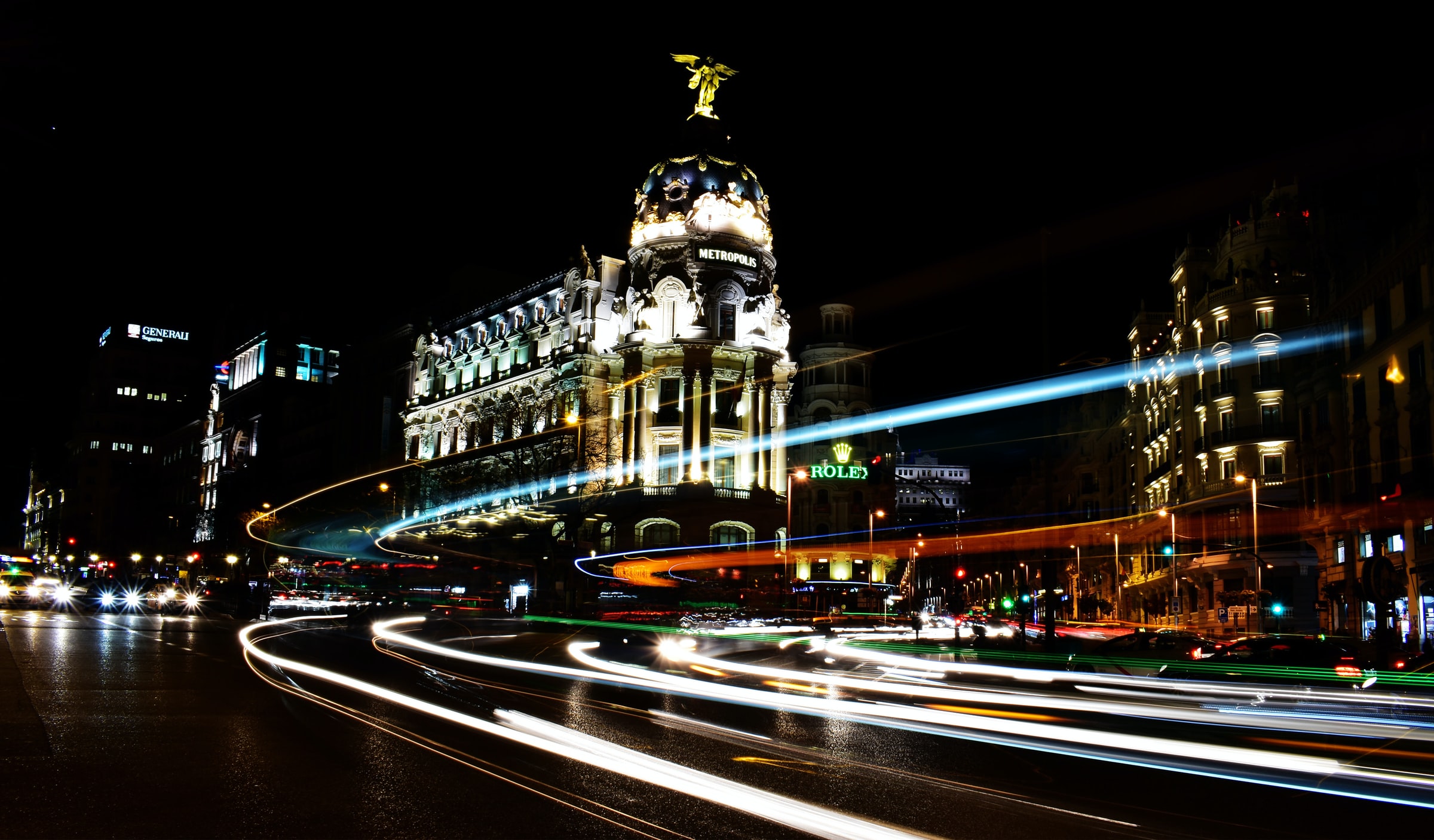
Madrid, a city with a strongly negative Average Concentration Index in the UESI. This means that the poor are disproportionately burdened by environmental issues such as air pollution and climate change, despite the overall strong performance on the indicators at the city level. Photo: Carlos Zurita, Unsplash.
Cities have emerged as important actors on the global sustainability stage. The UN’s Sustainable Development Goal-11 (SDG-11) sets the goal for cities to be sustainable and inclusive by 2030. As cities are centers of population growth and economic development worldwide, SDG-11 is critical because it is the first standalone urban goal in the UN system. Evidence has shown environmental challenges in cities such as air pollution and climate change are unevenly distributed, which is why it is so important that sustainable development is also inclusive, ensuring that the most marginalized communities are not left behind. Yet, setting targets does not create change in and of itself. Being able to track progress is a necessary first step towards holding decision-makers accountable to their targets.
Tracking global progress towards SDG-11 is challenging because it demands data that is comprehensive and spatially explicit. Comprehensive data, defined here as data that is available globally, is necessary so that all cities, both developed and developing, can participate in tracking their progress towards SDG-11. When cities independently decide on what indicators to use to measure SDG-11 progress, choosing the indicators that are most relevant to the unique conditions of that city often comes at the expense of comprehensive global data, since the indicators may not be measurable and meaningful across all cities. Spatially explicit data, which holds information on a fine-grain spatial scale such as a neighborhood, is important so we can track how different communities within a city are affected by environmental phenomena and policies. Spatially explicit data is especially important in cities, where much more diverse conditions can be found within a small area. However, collecting this type of data can be especially challenging for cities in the Global South, which lack well-funded and organized data collection institutions.
Organizations from the public and private sector have developed a number of urban sustainability indexes, for example the Global Liveable Cities Index and the Sustainable Cities Index, each of which compiles urban sustainability indicators to assess the performance of cities. But how useful are these indicators for measuring progress towards SDG-11? Data-Driven EnviroLab Principal Investigator Angel Hsu and Group Alumni Amy Weinfurter and Ryan Thomas investigated this question in their new paper in Environment and Planning: Urban Analytics and City Science.
Drawing from 40 indexes and online data repositories, they assessed the suitability of 484 existing urban sustainability indicators for measuring progress towards SDG-11’s call for cities to be sustainable and inclusive. Many indicators were unable to track SDG-11 progress because they lacked quantitative elements such as targets and baselines. One example of a specific target with a baseline is “reduce PM2.5 pollution levels by 25% by 2030 from 2000 levels” (target in blue, baseline in red). At the very least, targets should be directional if they do not have a baseline or clear target to be achieved—for instance, “reduce PM2.5 air pollution levels”. However, 57% of the assessed urban sustainability indicators did not have specific or directional targets, creating ambiguous management objectives. Most indicators also do not measure the equity component of SDG-11—only 13% of reviewed indicators are capable of measuring distributional equity (how sub-groups in a city are differently affected), and only 5% at the neighborhood scale.
With less than a decade to achieve the 2030 Agenda for Sustainable Development, policymakers need better ways of tracking progress towards SDG-11 to feed into their decision making. This is where the Urban Environment and Social Inclusion Index (UESI) comes in. Developed by Data-Driven EnviroLab, the UESI provides spatially-explicit indicators to measure environmental performance of 11,278 neighborhoods in 164 cities. The indicators measure a range of issue areas on a neighborhood-level scale, including air pollution, urban tree cover, public transport access, and Urban Heat Island. Water stress and climate mitigation are measured on the city-level.
The UESI uses publicly-available spatial datasets with global coverage and regular temporal resolution, as well as a transparent and reproducible methodology. This approach allows cities, especially underfunded governments in the Global South, to easily use UESI as a starting point for measuring progress towards SDG-11.
As outlined in a new paper in Frontiers in Sustainable Cities, the UESI indicated that 58% of cities disproportionately burden lower-income communities with higher shares of environmental burdens and lower shares of environmental benefits. Furthermore, although some cities such as Seattle, WA and Melbourne have overall strong performance on the environmental performance indicators, such as air pollution and water stress, their relatively poorer performance on the environmental inequality indicators show that overall higher performance does not necessarily lead to more equitable outcomes. This disparity affirms the need for a spatially-explicit focus when thinking about urban sustainability, as policies that are effective on the city level do not necessarily mean the conditions of the worst-affected communities equally benefit.
In partnership with the Samuel Centre for Social Connectedness, we will be working in the new year on the second phase of the UESI, which will entail including more cities as well as developing and improving upon our indicators. Specifically, we will be delving more into examining rural-urban air pollution gradients, expanding our analysis of considering unequal impacts of Urban Heat Island distributions, as well as incorporating land-use change metrics. If you’re interested in collaborating or contributing to this work, please get in touch.
Recent Comments